Data Topology is about spatial relationships and connectivity in data
Insights
Data Topology
Understanding and leveraging the structure of data is becoming increasingly crucial. This is where the concept of Data Topology, particularly as offered by Wingenium, comes into play. Wingenium's approach to data topology is not just innovative but also transformative, paving the way for new insights and solutions in various fields.
Data Topology, at its core, involves studying the shape and structure of data. It goes beyond mere numerical analysis, delving into how data points are arranged and related in multidimensional spaces. This approach allows us to see the 'big picture', revealing hidden patterns and connections that standard analyses might overlook.
Data Topology is concerned with the spatial relationships and connectivity within a dataset, unlike conventional methods that often simplify data structures, Data Topology retains the richness of intricate interconnections. It leverages techniques from algebraic topology, a branch of mathematics concerned with the properties of space preserved under continuous transformations.
How Wingenium Harnesses Data Topology
Wingenium's unique approach to Data Topology revolves around the sophisticated method of Topological Data Analysis (TDA). This technique is used to analyze high-dimensional data spaces, making it particularly suitable for handling complex, unstructured, and vast datasets.
Designing the Topological Framework
The first step in Wingenium's TDA process involves constructing a topological model of the data. This model is a representation of the data’s shape and structure. By abstracting data in this manner, Wingenium can identify features that are not immediately apparent.
Feature Extraction in TDA
Feature extraction in TDA is a critical process. It involves identifying shapes or structures within the data – such as loops, holes, or voids – and quantifying them. These features, known as topological invariants, are robust and can provide significant insights into the data's underlying patterns.
Using Betti Numbers for Insight
One of the key tools in TDA is the use of Betti numbers. These numbers count the number of n-dimensional holes in a dataset. For instance, Betti-0 represents the number of connected components, while Betti-1 counts the loops or cycles. These counts help in understanding the intrinsic properties of the data set.
Applying TDA to Machine Learning
The features extracted through TDA can be incredibly valuable for machine learning models. By incorporating these topological features, machine learning algorithms can achieve a deeper understanding of the data, leading to more accurate and robust models.
Enhanced Pattern Recognition
In machine learning, pattern recognition is crucial. Topological features provide a new dimension of data analysis, offering a more nuanced view of patterns and relationships within the data. This can be particularly beneficial in complex tasks like image recognition or anomaly detection.
Predictive Modeling
TDA can significantly enhance predictive modeling. By understanding the shape of data, models can make more accurate predictions, especially in scenarios where the data is highly dimensional or interconnected.
Practical Applications
The applications of Wingenium's data topology are vast and varied. In industries like healthcare, finance, and cybersecurity, the ability to uncover the underlying structure of data can lead to breakthroughs in prediction, analysis, and decision-making.
Case Study: Healthcare
In healthcare, for instance, TDA can help in understanding the complex relationships in genetic data, leading to better diagnostic tools and treatment plans.
Case Study: Finance
In finance, TDA can uncover subtle patterns in market data, offering traders and analysts a deeper understanding of market dynamics.
Wingenium's approach to Data Topology through TDA is more than just a technical methodology; it's a paradigm shift in how we understand and interact with data. By extracting and utilizing topological features, we can significantly enhance machine learning models, leading to more insightful, accurate, and predictive analytics. This revolutionary approach opens up new horizons in data analysis and application, promising exciting developments in various fields.
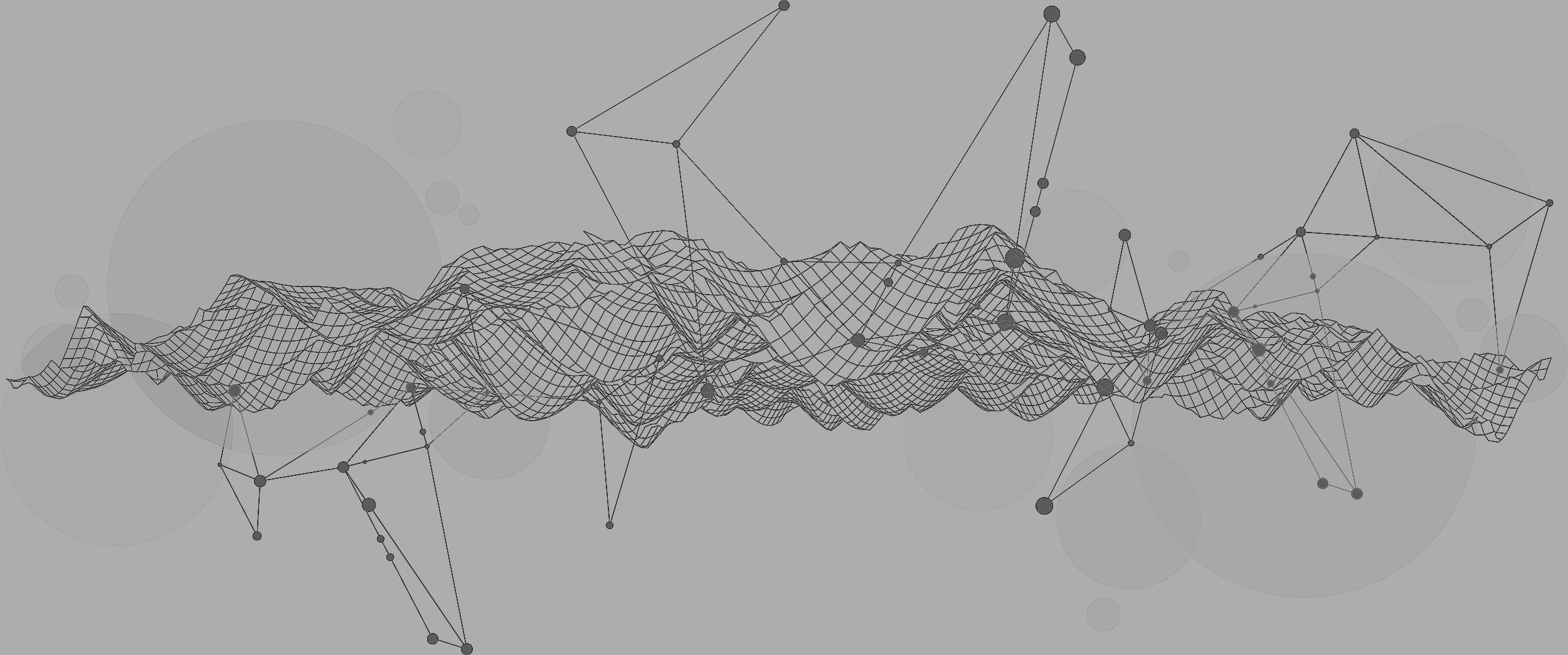
Would you like more information?
If you want to more information about this subject please get in touch with our Topology expert, who would be pleased to hear from you.
Invoke Ingenuity Data Topology Specialist
Ingenuity Framework is designed and maintained by our Data Topology team who are backed by our R&D on TDA sciences.
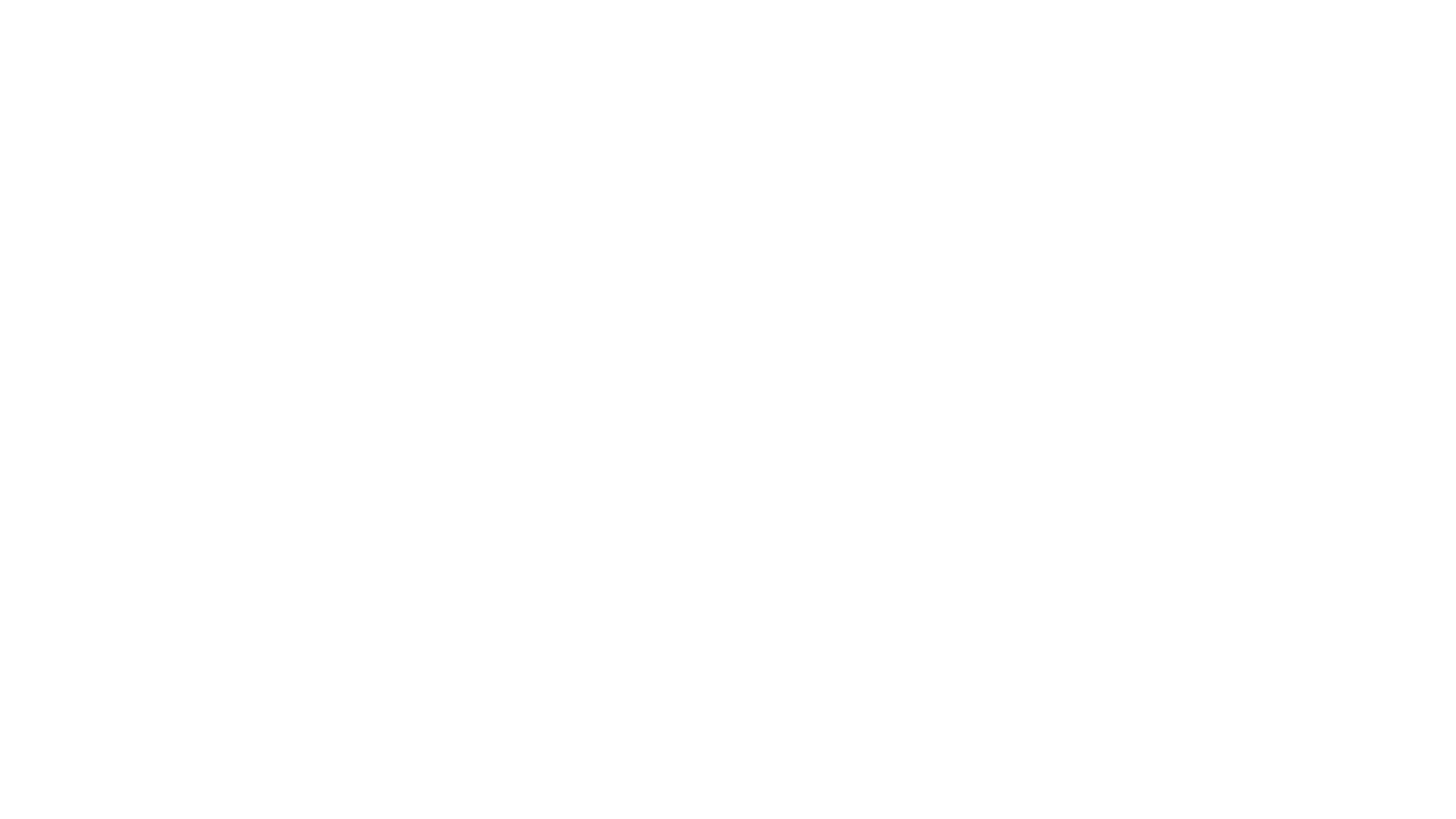